
Attentive Moment Retrieval in Videos
Abstract

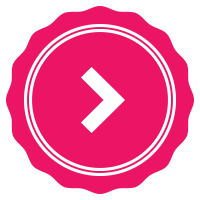
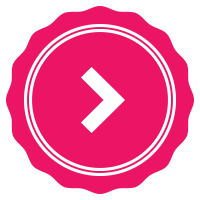
In the past few years, language-based video retrieval has attracted a lot of attention. However, as a natural extension, localizing the specific video moments within a video given a description query is seldom explored. Although these two tasks look similar, the latter is more challenging due to two main reasons: 1) The former task only needs to judge whether the query occurs in a video and returns an entire video, but the latter is expected to judge which moment within a video matches the query and accurately returns the start and end points of the moment. Due to the fact that different moments in a video have varying durations and diverse spatial-temporal characteristics, uncovering the underlying moments is highly challenging. 2) As for the key component of relevance estimation, the former usually embeds a video and the query into a common space to compute the relevance score. However, the later task concerns moment localization where not only the features of a specific moment matter, but the context information of the moment also contributes a lot. For example, the queries may contain temporal constraint words, such as ''first'', therefore need temporal context to properly comprehend them.
To address these issues, we develop an Attentive Cross-Modal Retrieval Network. In particular, we design a memory attention mechanism to emphasize the visual features mentioned in the query and simultaneously incorporate their context. In the light of this, we obtain the augmented moment representations. Meanwhile, a cross-modal fusion sub-network learns both the intra-modality and inter-modality dynamics, which can enhance the learning of moment-query representation. We evaluate our method on two datasets: DiDeMo and TACoS. Extensive experiments show the effectiveness of our model as compared to the state-of-the-art methods.
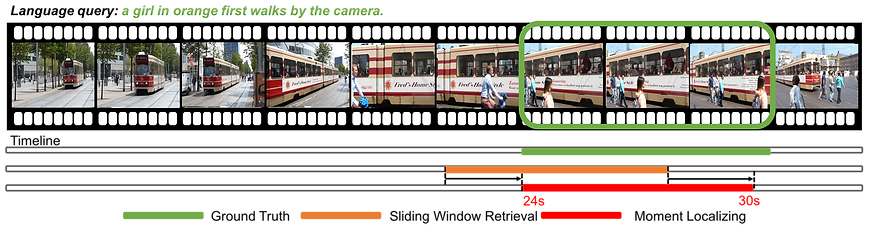

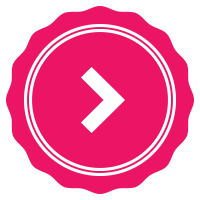
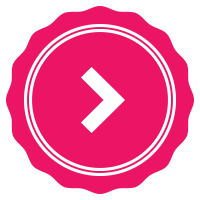
Model

Input: A set of moment candidates and the given query.
Output: A ranking model mapping each moment-query pair to a relevance score and estimating their location offsets of the golden moment.
As Figure illustrates, our proposed ACRN model comprises of the
following components:
1) The memory attention network leverages the weighting contexts to enhance the visual embedding of each moment;
2) The cross-modal fusion network explores the intra-modal and the inter-modal feature interactions to generate the moment-query representations;
3) The regression network estimates the relevance scores and predicts the location o sets of the golden moments.
Data & Codes

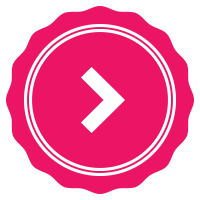
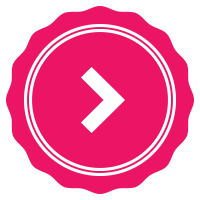
MCN:
TALL:
VSA-STV:
Glove:
Skip-thoughts:
VSA-RNN:
ACRN:
Examples

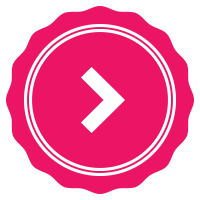
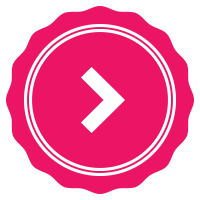
Here we show two examples, the left one from the DiDeMo dataset and the right one from the TACoS dataset. The red bounding box is our localization result, and the green box is the golden moment.
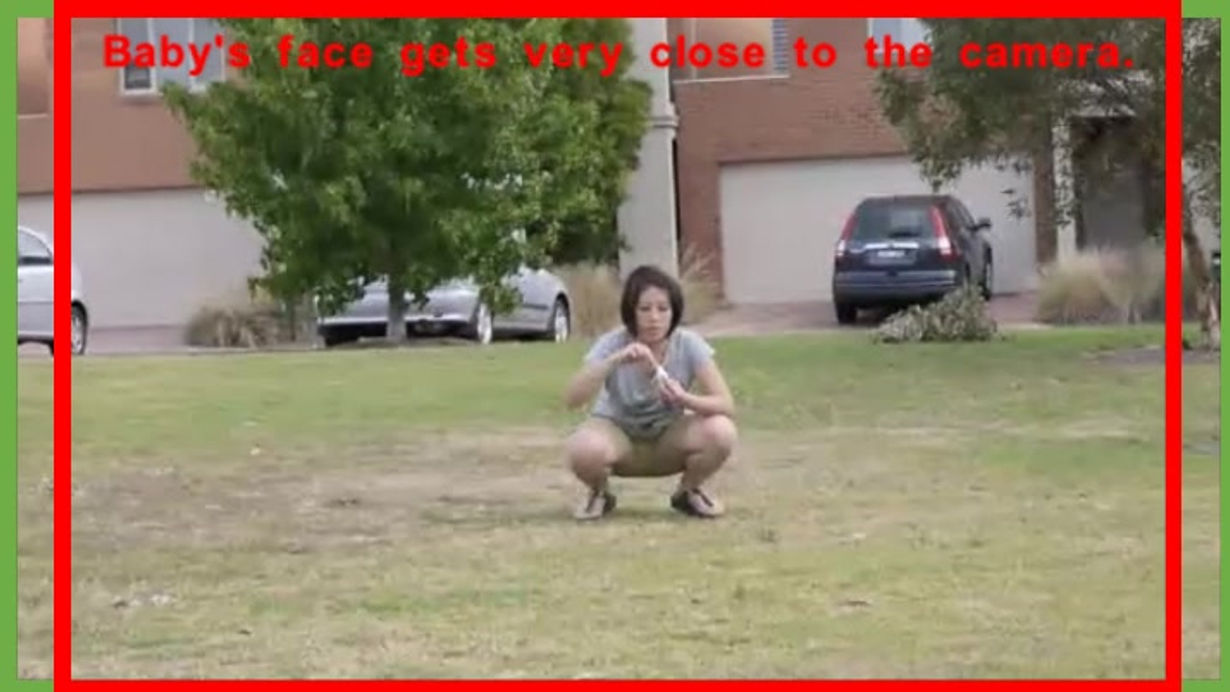
Example 1
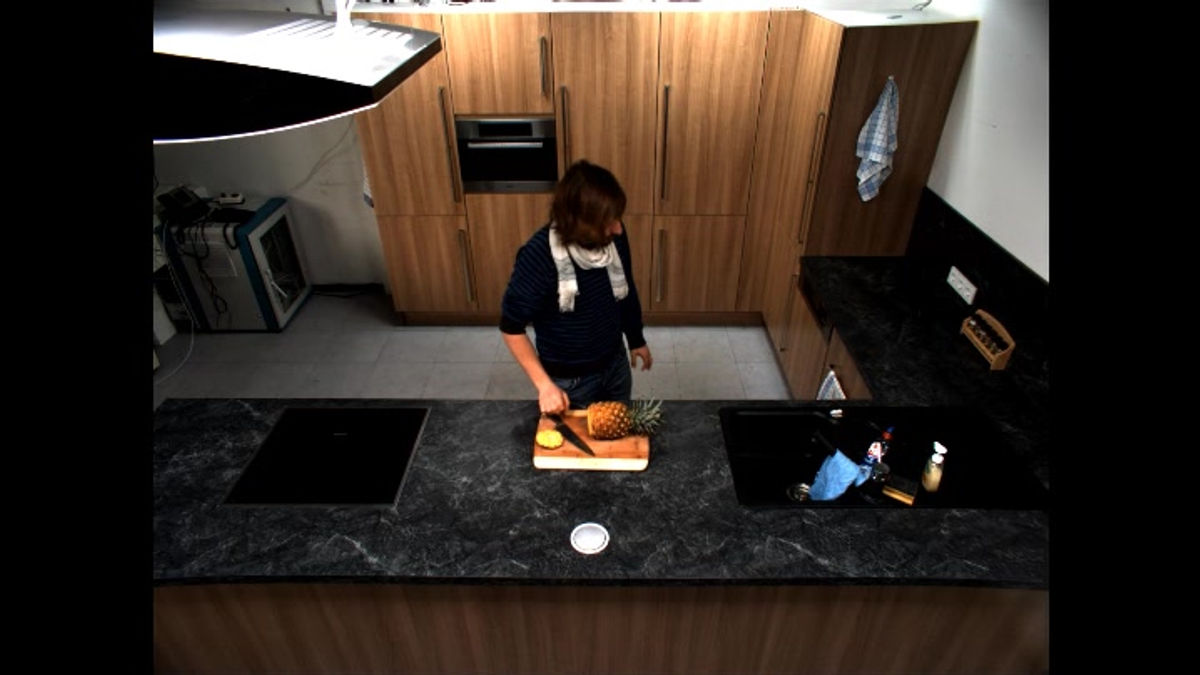